The population in South Africa is expected to keep growing a lot over the next few decades. To feed everyone, food production will need to increase too. However, the country already faces challenges with land and water shortages. Climate change is also making things harder with higher temperatures and less rain in some places. Traditionally, scientists did farm experiments to test things. But this takes a long time and money. They also only give results for one place and season. We need a faster way to get information to help farmers and make policies. Crop models on computers could help.
They let you quickly test different conditions without using real farms. Some challenges are the lack of good data for the models. They need detailed info on soils, weather, crops, and how farms are managed. However, trials in South Africa often don’t collect all the right data. Climate records and soil info may also be missing in some areas. Data is sometimes in the wrong format too. Farmers in dry summer rainfall areas will be hit hard by climate change. More reliable forecasts of seasons and future climate there are important. Crop models could help if we have the right data. We need to find better ways to get data for the models. Combining what we know with identifying gaps may help.
The goal is to run simulations easily to estimate climate change impacts and farming options. An integrated approach is needed to obtain suitable data meeting what the models require. This gives an overview of why we need crop models in South Africa and some of the challenges around data availability. Understanding these issues is the first step to using models to help with adaptation.
Key Takeaways
- Cost-Effective Data Collection: RS offers a cheaper alternative for collecting extensive agricultural data across large areas.
- Enhanced Model Accuracy: Integrating RS data improves the calibration and validation of crop simulation models, leading to more reliable predictions.
- Dynamic Data Integration: RS provides continuous updates that can be directly used to adjust and refine crop models in near-real time.
Disclaimer: Our article is a summary of the scientific document “Challenges and opportunities in crop simulation modeling under seasonal and projected climate change scenarios for crop production in South Africa”. The original version you can download HERE.
Remote Sensing in Crop Simulation
Remote sensed (RS) imagery can be used for mapping soil properties, classification of crop species, detection of crop water stress, monitoring of weeds and crop diseases, and mapping of crop yield. This technology offers substantial benefits for agricultural yield assessment and crop production modeling due to its high spatial, temporal, and spectral resolutions. Remote sensing data significantly enhances the accuracy of crop simulation models by providing up-to-date information on crop phenological stages critical for model accuracy. The integration of RS into crop simulation models allows for dynamic updates and calibration of model variables such as Leaf Area Index (LAI) and canopy nitrogen content, which are essential for predicting crop growth and yield.
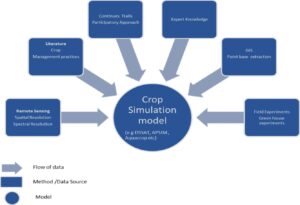
Synergy Between RS and Crop Models
Studies have shown that combining RS data with dynamic simulation models can lead to more accurate agricultural predictions and assessments. Techniques such as the ‘forcing’ strategy, where RS-derived observations are used to update state variables in models, and ‘run-time calibration,’ which adjusts model parameters in line with RS data, have proven effective. These integrations help in refining model outputs, particularly in terms of crop growth curves and phenological events, which are often challenging to predict accurately using traditional data sources alone.
Challenges and Opportunities
While RS provides a robust data source, there are challenges related to cloud cover and the availability of RS-derived parameters, which can affect the reliability of data used in crop models. However, these challenges can be mitigated through the use of complementary data collection methods and advanced processing techniques.
FAQ
What is Remote Sensing?
Remote Sensing involves collecting data from a distance, typically using satellites or aircraft, to observe and measure various characteristics of areas like agricultural fields.
How does RS benefit agriculture?
RS offers detailed, timely, and large-scale observations that help in efficient crop monitoring, management, and yield prediction.
Can RS data be integrated into all crop models?
Yes, RS data can be integrated into various crop models through techniques like model re-calibration and run-time data updates.
Summary
Using crop models to help farmers isn’t as easy as it sounds. There are a lot of challenges in getting all the right info the models need, like soil details, weather patterns, and how folks manage their land. Without good data, the models can’t give reliable answers. It seems in South Africa, the experiments scientists run don’t always collect everything needed. Weather records and soil tests are also missing in some places. Plus the data isn’t always in a format the models can use. This limits what research can be done to help crops. It’d be great to have the right info to show farmers the best planting times and practices for their specific areas.
Models could help reduce mistakes if we can improve the data situation. The good news is there are some ideas here for getting what we need! Combining available data sets from different sources might help fill in gaps. With work, we could get models calibrated to match local conditions better. More research on sharing data between models could also help. If one program can use what another collected, that’s a win. Overall it’s a complex issue but not impossible. With a team effort across fields, I think we can start testing these approaches on more crops. The goal is to use models to help folks adapt to climate change. With cooperation, we’ll get there!
Reference
Challenges and opportunities in crop simulation modelling under seasonal and projected climate change scenarios for crop production in South Africa Priscilla Ntuchu Kephe, K. Ayisi, B. Petja. Published in Agriculture & Food Security 8 April 2021. Agricultural and Food Sciences, Environmental Science. Kephe et al. Agric & Food Secur (2021) 10:10 https://doi.org/10.1186/s40066-020-00283-5.
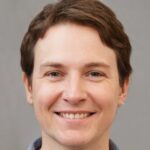
Hey guys! My name is Riley Burton and I love everything about the green world. Gardening is my passion – I find it so relaxing to get my hands in the dirt and help plants grow. In my free time you can usually find me outside exploring nature or writing about my gardening adventures. Hit me up if you ever want to learn more about the awesome world of plants!